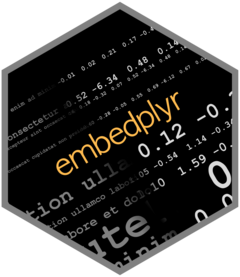
Find Nearest Tokens in Embedding Space
find_nearest.Rd
Find Nearest Tokens in Embedding Space
Usage
find_nearest(
object,
newdata,
top_n = 10L,
method = c("cosine", "euclidean", "minkowski", "dot_prod", "anchored"),
...,
each = FALSE,
include_self = TRUE,
decreasing = NULL,
get_sims = FALSE
)
Arguments
- object
an embeddings object made by
load_embeddings()
oras.embeddings()
- newdata
a character vector of tokens indexed by
object
, an embeddings object of the same dimensionality asobject
, or a numeric vector of the same dimensionality asobject
.- top_n
integer. How many nearest neighbors should be output? If
each = TRUE
, how many nearest neighbors should be output for each token?- method
either the name of a method to compute similarity or distance, or a function that takes two vectors and outputs a scalar, like those listed in Similarity and Distance Metrics. The value is passed to
get_sims()
.- ...
additional parameters to be passed to method function by way of
get_sims()
- each
logical. If
FALSE
(the default), the embeddings of newdata are averaged and the function will output an embeddings object with thetop_n
nearest tokens to the overall average embedding. IfTRUE
, the function will output a named list with one embeddings object for each token in newdata.- include_self
logical. Should the token(s) in
newdata
be included in the output?- decreasing
logical. Determines the order of sorting the similarity scores. If
TRUE
(the default for"cosine"
,"dot_prod"
, and"anchored"
methods), the embeddings with the highest similarity scores are output. IfFALSE
(the default for"euclidean"
and"minkowski"
methods), the embeddings with the lowest distance scores are output.- get_sims
logical. If
FALSE
(the default), output an embeddings object or list of embeddings objects. IfTRUE
, output a tibble with similarity or distance scores for each document.
Value
If get_sims = FALSE
(the default), an embeddings object or list of
embeddings objects. If get_sims = TRUE
, a tibble or list of tibbles
with similarity or distance scores for each document, with columns doc_id
and the name of the requested method
.
Examples
words <- c("happy", "sad")
words_embeddings <- predict(glove_twitter_25d, words)
find_nearest(glove_twitter_25d, words)
#> # 25-dimensional embeddings with 10 rows
#> dim_1 dim_2 dim_3 dim_4 dim_5 dim_6 dim_7 dim_8 dim_9 dim..
#> miss -0.65 0.59 0.36 0.01 -1.05 -0.02 1.34 1.46 -0.84 -0.14 ...
#> happy -1.23 0.48 0.14 -0.03 -0.65 -0.19 2.10 1.75 -1.30 -0.32 ...
#> love -0.63 -0.08 0.07 0.58 -0.87 -0.15 2.23 0.99 -1.32 -0.35 ...
#> wish -0.52 1.12 0.49 -0.25 -1.01 0.38 2.00 0.56 -0.56 0.17 ...
#> you -0.42 0.33 -0.09 0.20 -0.80 -0.34 2.14 0.37 -0.94 0.24 ...
#> thank -0.90 0.70 -0.06 -0.03 -0.77 -0.95 2.04 0.53 -1.30 0.07 ...
#> sad 0.04 -0.19 0.44 -0.15 -0.60 0.05 1.47 0.14 -0.72 0.43 ...
#> too -0.47 0.40 0.12 -0.00 -0.21 0.46 1.68 0.08 -0.77 0.08 ...
#> good -0.54 0.60 -0.15 -0.02 -0.14 0.60 2.19 0.21 -0.52 -0.23 ...
#> hope -0.77 0.81 0.02 -0.23 -1.28 -0.31 1.83 0.19 -0.86 -0.23 ...
find_nearest(glove_twitter_25d, words_embeddings) # equivalent to previous
#> # 25-dimensional embeddings with 10 rows
#> dim_1 dim_2 dim_3 dim_4 dim_5 dim_6 dim_7 dim_8 dim_9 dim..
#> miss -0.65 0.59 0.36 0.01 -1.05 -0.02 1.34 1.46 -0.84 -0.14 ...
#> happy -1.23 0.48 0.14 -0.03 -0.65 -0.19 2.10 1.75 -1.30 -0.32 ...
#> love -0.63 -0.08 0.07 0.58 -0.87 -0.15 2.23 0.99 -1.32 -0.35 ...
#> wish -0.52 1.12 0.49 -0.25 -1.01 0.38 2.00 0.56 -0.56 0.17 ...
#> you -0.42 0.33 -0.09 0.20 -0.80 -0.34 2.14 0.37 -0.94 0.24 ...
#> thank -0.90 0.70 -0.06 -0.03 -0.77 -0.95 2.04 0.53 -1.30 0.07 ...
#> sad 0.04 -0.19 0.44 -0.15 -0.60 0.05 1.47 0.14 -0.72 0.43 ...
#> too -0.47 0.40 0.12 -0.00 -0.21 0.46 1.68 0.08 -0.77 0.08 ...
#> good -0.54 0.60 -0.15 -0.02 -0.14 0.60 2.19 0.21 -0.52 -0.23 ...
#> hope -0.77 0.81 0.02 -0.23 -1.28 -0.31 1.83 0.19 -0.86 -0.23 ...
find_nearest(glove_twitter_25d, words, each = TRUE)
#> $happy
#> # 25-dimensional embeddings with 10 rows
#> dim_1 dim_2 dim_3 dim_4 dim_5 dim_6 dim_7 dim_8 dim_9
#> happy -1.23 0.48 0.14 -0.03 -0.65 -0.19 2.10 1.75 -1.30 ...
#> birthday -1.46 0.50 1.00 0.17 -0.68 -0.75 1.82 1.55 -1.24 ...
#> thank -0.90 0.70 -0.06 -0.03 -0.77 -0.95 2.04 0.53 -1.30 ...
#> welcome -0.97 0.88 -0.25 -0.54 -0.55 -0.44 1.46 0.78 -0.70 ...
#> love -0.63 -0.08 0.07 0.58 -0.87 -0.15 2.23 0.99 -1.32 ...
#> miss -0.65 0.59 0.36 0.01 -1.05 -0.02 1.34 1.46 -0.84 ...
#> hello -0.77 0.13 0.33 0.01 -0.48 -0.50 1.86 1.06 -0.57 ...
#> thanks -0.80 0.82 -0.28 -0.11 -0.55 -0.72 1.62 0.98 -1.00 ...
#> merry -1.23 0.56 0.37 0.41 -0.56 -0.65 1.62 0.36 -1.62 ...
#> bless -1.15 0.81 -0.26 0.77 -0.68 -0.82 1.40 0.55 -1.23 ...
#>
#> $sad
#> # 25-dimensional embeddings with 10 rows
#> dim_1 dim_2 dim_3 dim_4 dim_5 dim_6 dim_7 dim_8 dim_9
#> sad 0.04 -0.19 0.44 -0.15 -0.60 0.05 1.47 0.14 -0.72 ...
#> feel -0.33 0.07 0.15 -0.17 -0.26 0.94 2.28 -0.17 -1.04 ...
#> same 0.53 0.30 0.47 -0.02 -0.00 0.33 1.30 -0.05 -0.15 ...
#> wrong 0.68 0.45 0.26 0.38 -0.06 -0.02 1.36 -0.64 -0.65 ...
#> meant 0.22 0.20 0.17 -0.26 -0.68 -0.37 1.32 -0.92 -0.92 ...
#> true 0.40 0.02 -0.63 0.04 -0.01 0.11 1.84 0.55 -0.94 ...
#> reason 0.44 0.42 -0.00 0.33 -0.09 0.02 1.87 -0.57 -0.62 ...
#> remember 0.12 0.77 0.56 0.20 -0.91 -0.26 1.93 -0.02 -0.87 ...
#> i -0.26 0.59 0.62 -0.70 -0.85 -0.23 1.05 0.07 -0.55 ...
#> know 0.30 0.70 -0.00 -0.06 -0.69 -0.14 1.95 0.01 -0.51 ...
#>
find_nearest(glove_twitter_25d, words_embeddings, each = TRUE) # equivalent to previous
#> $happy
#> # 25-dimensional embeddings with 10 rows
#> dim_1 dim_2 dim_3 dim_4 dim_5 dim_6 dim_7 dim_8 dim_9
#> happy -1.23 0.48 0.14 -0.03 -0.65 -0.19 2.10 1.75 -1.30 ...
#> birthday -1.46 0.50 1.00 0.17 -0.68 -0.75 1.82 1.55 -1.24 ...
#> thank -0.90 0.70 -0.06 -0.03 -0.77 -0.95 2.04 0.53 -1.30 ...
#> welcome -0.97 0.88 -0.25 -0.54 -0.55 -0.44 1.46 0.78 -0.70 ...
#> love -0.63 -0.08 0.07 0.58 -0.87 -0.15 2.23 0.99 -1.32 ...
#> miss -0.65 0.59 0.36 0.01 -1.05 -0.02 1.34 1.46 -0.84 ...
#> hello -0.77 0.13 0.33 0.01 -0.48 -0.50 1.86 1.06 -0.57 ...
#> thanks -0.80 0.82 -0.28 -0.11 -0.55 -0.72 1.62 0.98 -1.00 ...
#> merry -1.23 0.56 0.37 0.41 -0.56 -0.65 1.62 0.36 -1.62 ...
#> bless -1.15 0.81 -0.26 0.77 -0.68 -0.82 1.40 0.55 -1.23 ...
#>
#> $sad
#> # 25-dimensional embeddings with 10 rows
#> dim_1 dim_2 dim_3 dim_4 dim_5 dim_6 dim_7 dim_8 dim_9
#> sad 0.04 -0.19 0.44 -0.15 -0.60 0.05 1.47 0.14 -0.72 ...
#> feel -0.33 0.07 0.15 -0.17 -0.26 0.94 2.28 -0.17 -1.04 ...
#> same 0.53 0.30 0.47 -0.02 -0.00 0.33 1.30 -0.05 -0.15 ...
#> wrong 0.68 0.45 0.26 0.38 -0.06 -0.02 1.36 -0.64 -0.65 ...
#> meant 0.22 0.20 0.17 -0.26 -0.68 -0.37 1.32 -0.92 -0.92 ...
#> true 0.40 0.02 -0.63 0.04 -0.01 0.11 1.84 0.55 -0.94 ...
#> reason 0.44 0.42 -0.00 0.33 -0.09 0.02 1.87 -0.57 -0.62 ...
#> remember 0.12 0.77 0.56 0.20 -0.91 -0.26 1.93 -0.02 -0.87 ...
#> i -0.26 0.59 0.62 -0.70 -0.85 -0.23 1.05 0.07 -0.55 ...
#> know 0.30 0.70 -0.00 -0.06 -0.69 -0.14 1.95 0.01 -0.51 ...
#>
rand_vec <- rnorm(25)
find_nearest(glove_twitter_25d, rand_vec)
#> # 25-dimensional embeddings with 10 rows
#> dim_1 dim_2 dim_3 dim_4 dim_5 dim_6 dim_7 dim_8
#> entertaining 0.23 0.27 0.07 -0.65 0.83 1.09 1.24 -1.42 ...
#> nervous -0.45 0.17 0.21 -0.80 0.24 0.89 1.91 -0.57 ...
#> relaxed -1.93 -0.55 -0.63 -0.43 0.51 2.03 0.29 -1.48 ...
#> enjoyable -0.66 0.07 -0.23 -0.63 0.48 1.29 1.63 -1.73 ...
#> surprisingly -0.62 -0.56 0.19 -1.03 0.09 1.68 0.99 -1.63 ...
#> instructors -0.74 0.91 -0.14 -1.04 0.85 -0.20 -0.08 -1.92 ...
#> routines -0.21 0.42 -0.04 -0.30 1.11 0.45 0.69 -2.46 ...
#> productive -0.85 0.68 -0.40 -0.81 0.97 1.20 1.38 -1.74 ...
#> philosophical -0.43 0.11 -0.77 0.93 1.00 1.50 1.02 -1.77 ...
#> sgt 0.47 -0.89 -0.43 -1.23 -0.24 2.14 -0.43 1.63 ...